Running a help desk is no easy task. Supervisors spend countless hours listeningto calls, spotting errors, and identifying ways for agents to improve.Yet, despite all this effort, mistakes still happen, and opportunities toenhance service often slip by. Without real-time feedback, making meaningful,on-the-spot changes becomes a challenge—leaving both agents and customersfrustrated.This is where AI steps in. Tools like speech-to-text technology and GPT-poweredsentiment analysis eliminate much of the guesswork. These solutionsautomatically review calls, flag issues, and offer actionable insights, allowingteams to focus on what truly matters: helping customers. Instead of siftingthrough hours of recordings, supervisors gain access to accurate, real-timeevaluations and practical suggestions for improvement. The result? Fasterresponses, more consistent service, and happier customers.According to Gartner,by 2025, 80% of customer service organizations will leverage generative AItechnology to boost agent productivity and enhance the customer experience (CX).This growing reliance on AI reflects its potential to transform customer supportoperations.In this article, we’ll dive into how these AI-powered tools work and how businesses are already using them totransform customer support.WHAT ARE SPEECH-TO-TEXT AND GPT SENTIMENT ANALYSIS?At its core, speech-to-text technology does exactly what the name suggests: itconverts spoken language into written text. Whether it’s a live conversation ora recorded call, the technology listens, transcribes, and organizes what wassaid in a format that’s easy to analyze. This automation eliminates the need formanual note-taking or transcription, saving time and ensuring accuracy. Manyspeech-to-text systems now use LLMs to better handle differentaccents, speech patterns, and industry-specific terminology.GPT-powered sentiment analysis goes a step further by interpreting the emotionaltone and context of those conversations. It analyzes words, phrases, and evensubtle cues to understand whether a customer is satisfied, frustrated, orconfused. This real-time emotional insight helps businesses respond moreeffectively, whether it’s resolving an issue or identifying areas forimprovement.Generative AI tools, like those behind GPT analysis, deliver measurableimprovements. For example,57%of companies using these technologies report better customer effort scores,while 56% see higher agent productivity.Deloitte Digital 2024Together,these technologies are a powerhouse for improving customer support.Speech-to-text captures the “what” of a conversation, while sentiment analysisinterprets the “how.” This combination provides a deeper understanding ofcustomer interactions, enabling businesses to spot trends, refine processes, andcreate experiences that keep customers coming back.REAL-WORLD USE CASES TRANSFORMING HELPDESK OPERATIONSGenerative AI is increasingly becoming a cornerstone of modern customer support,helping teams streamline workflows and deliver faster responses. In fact,according to HubSpot’s State of AI report, service professionalssave more than two hours a day by using generative AI to respond to customersquickly.USE CASE 1: BLUEDOT ENHANCING SALES PERFORMANCESales teams often struggle to pinpoint what makes their best calls successful.Manual reviews are time-consuming, and key insights can easily be overlooked. Totackle this, leverages Bluedot , anAI-powered tool that combines speech-to-text and sentiment analysis to enhancecall evaluations and improve sales outcomes. This significantly reduces meetingdocumentation time—from 1–2 days to just 5–10 minutes—while providing instantaccess to call summaries and action items.Here’s how it works: Bluedot records calls directly from platforms like GoogleMeet or Zoom. These calls are automatically transcribed and synced with HubSpot,eliminating the need for manual note-taking. Beyond transcription, Bluedotanalyzes the tone and content of each call, providing summaries that highlightcritical moments, such as how objections were handled or how product benefitswere communicated.The results? Sales teams gain instant insights into what works. For example,Bluedot might identify that successful calls emphasize a specific feature or usecertain phrasing that resonates with clients. These insights help refine salesscripts and strategies, leading to improved performance across the board.Additionally LLMs add another layer by offering feedback oncommunication—highlighting clarity, tone, and key areas for improvement, such asphrasing or objection handling.USE CASE 2: INSURANCE HELPDESK – POC BY R&D R&D team developed a proof-of-concept (PoC) system that leverages speech-to-text technologyand GPT analysis to optimize insurance help desk operations.Here’s how it works: The system transcribes call audio into text, handling evenlengthy conversations exceeding 20 minutes. Once transcribed, GPT analyzes thecontent to assess whether the agent followed company best practices, effectivelyaddressed customer needs, and complied with regulatory guidelines.The system also generates concise summaries, highlighting key moments andidentifying any deviations from standards, such as compliance requirements orinternal policies. Supervisors receive real-time feedback on agent performance,making it easier to pinpoint issues and implement corrective actions quickly.During testing, the PoC successfully analyzed insurance claims calls, deliveringdetailed compliance reports and practical recommendations to improve agentadherence to regulations and customer service standards.USE CASE 3: TECHNICAL SUPPORT – FASTER TROUBLESHOOTINGIn technical support, agents must quickly diagnose issues and follow stricttroubleshooting protocols. A speech-to-text system paired with GPT analysiscould ease this process by transcribing calls, verifying adherence toguidelines, and identifying recurring problems.For instance, in a banking scenario, customers often call about issues likefailed online payments or trouble accessing their accounts. If the systemdetects a recurring problem—such as login errors following a recent systemupdate—it can flag the issue for escalation to the IT team. Meanwhile, agentscan rely on the tool to provide consistent and accurate guidance, such aswalking customers through password resets or suggesting alternativeauthentication methods.USE CASE 4: RETAIL AND COMMERCE – IMPROVING CUSTOMER INTERACTIONSA speech-to-text system with GPT analysis could help monitor customer support calls to ensure agentsconsistently follow return, exchange, and inquiry-handling policies. The systemmight pinpoint recurring issues—such as customers misunderstanding refundtimelines—and provide actionable insights to improve service protocols andcommunication.For example, if multiple calls reveal confusion over return eligibility,businesses could refine their policies and train agents to explain the processmore effectively. Additionally, the system could analyze frequent after-salesinquiries, offering proactive solutions like automated follow-up emails withproduct care tips or shipping updates to address concerns before they arise.USE CASE 5: E-COMMERCE – POST-PURCHASE SUPPORTA speech-to-text system with GPT analysis can help e-commerce businesses enhancepost-purchase support by ensuring agents consistently handle complaints,returns, and inquiries. By analyzing conversations, the system verifies thatagents adhere to standardized processes, such as refund timelines or replacementpolicies, across all interactions.For instance, if the system detects inconsistencies in how agents resolvesimilar complaints, it can flag these discrepancies and recommend targetedtraining to maintain uniform service quality. Additionally, the system couldidentify recurring post-purchase issues—like frequent complaints about delayeddeliveries—enabling businesses to proactively address underlying problems andimprove the customer experience.SUMMARY OF SPEECH-TO-TEXT WITH GPT SENTIMENT ANALYSIS USE CASESUse CaseKey ChallengesAI’s RoleOutcomesSales PerformanceUnderstanding successful sales strategiesAutomates call reviews, analyzes tone and content, highlights key momentsRefines sales scripts, improves pitch deliveryInsurance HelpdeskEnsuring compliance and service qualityTranscribes and evaluates calls for adherence to regulationsProvides real-time feedback, improves compliance, and reduces manual review timeTechnical SupportDiagnosing and resolving issues quicklyIdentifies recurring problems, verifies adherence to troubleshooting protocolsReduces resolution times, improves operational efficiencyRetail and CommerceConsistent handling of customer inquiriesMonitors adherence to policies and identifies trends in common complaintsRefines service protocols, improves communicationPost-PurchaseMaintaining consistency in support qualityTracks agent consistency, highlights recurring issuesStreamlines post-purchase support, resolves complaints fasterREADY-TO-USE SPEECH-TO-TEXT WORKFLOWS: HOW THEY WORKIntegrating speech-to-text capabilities into helpdesk operations is moreefficient with pre-built AI solutions, removing the need to develop custommodels. These tools provide APIs and SDKs that allow businesses to incorporateadvanced speech recognition and voice processing intotheir workflows with minimal setup. At , we often use OpenAI Whisper andMicrosoft Azure Speech Services to build custom solutions for our clients.OPENAI WHISPER: MULTILINGUAL, CONTEXT-AWARE SPEECH RECOGNITIONWhisper is OpenAI’s automatic speechrecognition (ASR) model designed for high-accuracy transcription in multiplelanguages. Unlike traditional speech recognition systems, Whisper is trained onvast multilingual speech data, enabling it to transcribe speech in multiplelanguages and translate non-English content into English.It excels in handling complex audio conditions, such as background noise,accents, and overlapping speech. Using a transformer-based neural network, itmaps audio waveforms to text sequences while refining predictions in real time.Open AI Whisper speech-to-text workflow screenshotSource: OpenAI.com MICROSOFT AZURE SPEECH SERVICES: ENTERPRISE-GRADE SPEECH-TO-TEXT &TEXT-TO-SPEECHAzure AI Speech providesscalable speech-to-text and text-to-speech services that integrate seamlesslyinto enterprise workflows. Its speech-to-text API uses deep neural networks totranscribe spoken language into structured text, offering features like speakerdiarization and automatic punctuation for improved readability.Microsoft Azure Speech Services website screenshotSource:ai.azure.com TWILIO SPEECH RECOGNITION: REAL-TIME AI-POWERED TRANSCRIPTIONTwilio provides real-time speech recognition APIsthat transcribe voice interactions instantly. Its ASR models process live audio,analyzing phonemes (smallest units of sound) and predicting words with highaccuracy. This enables instant transcription, real-time conversation monitoring,and sentiment analysis for customer service teams.TwilioSource: Twilio GOOGLE CLOUD SPEECH-TO-TEXT: SCALABLE AI-POWERED TRANSCRIPTIONGoogle Cloud providesspeech-to-text and text-to-speech services designed for high scalability andreal-time applications. Its speech-to-text API supports over 125 languages andis optimized for various industries, including customer service, healthcare, andfinance.Google’s deep learning models offer speaker diarization, automatic punctuation,and domain adaptation, allowing businesses to fine-tune models forindustry-specific terminology. It also provides batch processing and real-timestreaming, making it effective for both live and recorded customer interactions.Google CloudSource:cloud.google.com CHALLENGES AND LIMITATIONS OF SPEECH-TO-TEXT WITH GPT ANALYSISWhile speech-to-text and GPT analysis offer significant benefits, implementingthese technologies comes with challenges.One of the main AI concerns is data privacy and security,as handling customer conversations requires strict compliance with regulationslike GDPR or CCPA. Sensitive information, such as payment details or personalidentifiers, must be protected during transcription and analysis. Businesses canmitigate risks using encryption and anonymization techniques.Additionally, the EU AI Act classifies AI systems processingsensitive customer data as high-risk, requiring stricter compliance measuressuch as transparency, risk assessments, and human oversight. For example, afinancial institution might implement end-to-end encryption to safeguardcustomer account information while still leveraging AI insights from calltranscripts.OpenAI Whisper is highly effective in handling background noise, strong accents,and multilingual support, making it well-suited for call centers and globalhelpdesk operations. However, accuracy declines when multiple speakers overlapor when the conversation involves technical jargon outside the model’s trainingdata.Microsoft Azure Speech Services provides enterprise-grade transcription andindustry-specific adaptation, but like all ASR models, it can struggle with pooraudio quality, specialized terminology, or complex legal and medical contexts.In high-stakes environments where precision is crucial, human oversight isessential to validate and refine transcripts for compliance and accuracy.Google Cloud Speech-to-Text allows custom model tuning for specific industrieslike finance, healthcare, and customer service. While this customizationimproves accuracy for domain-specific language, implementation requiresadditional data training and continuous fine-tuning.Despite these capabilities, accuracy limitations remain, especially inchallenging environments or conversations with multiple speakers. While AImodels continue to improve ,human oversight is still necessary in edge cases to validate and refine resultswhere precision is crucial.ENSURING ACCURACY IN AI-POWERED TRANSCRIPTIONAccuracy is critical in AI transcription for customer-facing roles, as errorscan damage trust and cause issues, especially in regulated industries likehealthcare. OpenAI’s Whisper, a speech-to-text model used by hospitals throughNabla’s platform, has reportedly processedover 9 million medical conversations for more than 30,000 clinicians and 40health systems. While Nabla has stated that hallucination errors are rare intheir use case, the tool’s limitations are well-documented.For example, research from Cornell University and the University of Washingtonfound thatapproximately 1% of transcriptions generated by Whisper contained fabricatedcontent, with 38% of those inaccuracies leading to potential harm ormisrepresentation. Such errors were more frequent in conversations with extendedpauses, particularly those involving patients with speech disorders likeaphasia.To address these risks, Nabla incorporates a secondary validation process,cross-checking AI-generated transcripts against verified records to ensure onlyaccurate information is used. This highlights the importance of combiningautomation with human oversight, particularly for businesses adopting AItranscription tools in high-stakes or customer-facing contexts.Beyond human oversight, additional AI techniques can further enhancetranscription accuracy. For example, “word healing” leverages statisticalprobabilities to correct common grammatical errors—ensuring “I am” is recognizedinstead of “I is.” Generative AI can refinetranscripts by structuring sentences more naturally, reducing inconsistenciescaused by misinterpretation.Voice quality also plays a key role in improving transcription results. Noisereduction techniques, such as low-pass filtering, can isolate speech frombackground noise by prioritizing louder voices. More advanced solutions, likemachine learning models designed to “clean” audio files, can further enhanceclarity by filtering out unwanted sounds.By combining AI-driven corrections, noise reduction, and human validation,businesses can reduce hallucinations and improve transcription accuracy.INTEGRATION WITH EXISTING TOOLS: CHALLENGES AND CONSIDERATIONS 1. Data Flow and Accessibility * Streamlined Data Pipelines: Many contact centers rely on legacy systems or multiple databases (CRM, ticketing tools, analytics platforms). Ensuring that call transcripts and sentiment scores flow seamlessly between systems requires careful planning of data transfer protocols (e.g., APIs, webhooks) and data mapping. * Data Sync vs. Real-Time Processing: Depending on business needs, data can be processed in batches (post-call) or in near-real-time (during the call). Integrations must accommodate the required speed, which can impact your choice of architecture and technology stack. 2. Compliance and Security * Regulatory Requirements: For industries such as healthcare or finance, integrations must respect standards like GDPR, CCPA, HIPAA, or PCI-DSS. This often entails encryption, anonymization, and careful handling of sensitive transcripts. * Authentication and Authorization: Using single sign-on (SSO) or role-based access control (RBAC) can protect sensitive customer data. Ensure that each tool in the workflow enforces the same security protocols. * Audit Trails: Implement logging and audit capabilities to track how data moves through systems, who accesses it, and what changes are made. This is crucial for regulatory audits and internal compliance. 3. Compatibility and Customization * Vendor Lock-In: Some third-party AI or transcription services may limit how easily you can switch providers or add new functionalities. Look for tools offering flexible APIs and broad integration capabilities. * Custom Vocabulary and Domain-Specific Models: Businesses in specialized domains (e.g., insurance, medicine, manufacturing) may need custom language models to achieve high accuracy. Integrations should support training or uploading custom vocabularies for niche terminology. * Version Control and Upgrades: AI services frequently update models. Plan for how your integrated system will handle new features, version changes, or API deprecations without disrupting service. 4. Operational Workflow Adjustments * Agent and Supervisor Adoption: Even the best AI integration can fail if end users resist or find the tools cumbersome. Provide training, user-friendly dashboards, and clear onboarding processes to ensure agent and supervisor buy-in. * UI/UX Consistency: If sentiment scores or transcriptions appear in a CRM, ensure the user interface is intuitive. Align visual elements, language, and navigation with existing tools so users have a unified experience. * Notifications and Alerts: Determine how and when the system alerts supervisors or agents about potential issues—e.g., non-compliance, negative customer sentiment. Real-time pop-ups vs. daily summary reports can dramatically affect how quickly problems are resolved. 5. Scalability and Performance * Concurrent Call Handling: Large contact centers may handle thousands of calls simultaneously. Integrations should be load-tested to ensure stable performance under peak volumes. * Cloud Infrastructure vs. On-Premises: Decide if a fully cloud-based solution is viable or if on-premises or hybrid setups are necessary for privacy or compliance. The choice affects cost, scalability, and latency. * Monitoring and Resilience: Use monitoring tools (e.g., Grafana, Kibana, or built-in dashboards from cloud services) to track latency, error rates, and resource usage. Implement fallback mechanisms, such as queueing or secondary transcription services, to handle outages or spikes. 6. Cost Management * Licensing and Subscription Fees: Speech-to-text and GPT APIs often charge based on usage (minutes of audio, tokens processed, or monthly active agents). Monitor these metrics to prevent unexpected costs. * Hidden Integration Costs: Beyond API fees, factor in data storage, network egress (if large audio files are transferred), and additional developer time for maintenance and troubleshooting. * ROI Measurement: Continuously measure time saved in manual call reviews, improvement in compliance, or reductions in handling time. Benchmarking these improvements helps justify ongoing expenses. 7. Testing and Iterative Rollouts * Proof of Concept (PoC) First: Start by integrating a small subset of calls or a single department to validate the solution’s effectiveness and discover pain points early. * A/B Testing: Compare the AI-enhanced process against the existing workflow to quantify improvements in agent performance, customer satisfaction, or first-call resolution rates. * Feedback Loops: Gather input from agents, supervisors, and customers. Use their insights to fine-tune integrations, improve model accuracy, and refine the user experience.Speech-to-Text with GPT Analysis Integration AspectsHOW TO GET STARTED WITH SPEECH-TO-TEXT + GPT ANALYSISImplementing speech-to-text and GPT analysis can transform customer support, buta structured approach is key to success. Here’s how to get started: * Identify pain points and objectives: Assess your current challenges—such as lengthy call reviews or inconsistent service quality—and define clear goals, like improving call handling or enhancing agent training. * Choose the right technology stack: Evaluate available tools for speech-to-text transcription and GPT analysis. Look for options that align with your objectives and can integrate with your existing systems. * Develop and test a proof of concept (PoC): Design a small-scale implementation. Test it in real-world scenarios to evaluate its performance and identify areas for refinement. * Scale based on feedback and results: Use insights from the PoC to fine-tune the system and roll it out across larger teams or departments. Monitor its impact and continuously improve based on feedback.TECHNICAL CONSIDERATIONS FOR IMPLEMENTATIONTo successfully integrate speech-to-text and GPT-powered analysis, businessesneed to plan forscalability, security, and adoption: * Plan for Architecture – Decide whether to process calls in real-time or batches, as this choice affects integration complexity and system performance. * Prioritize Security and Compliance – Implement encryption, anonymization, and strict access controls to safeguard sensitive customer data. * Ensure Scalability – AI transcription and sentiment analysis can be CPU/GPU-intensive. Prepare to scale infrastructure or use cloud-based solutions to handle peak demand. * Foster User Adoption – Design intuitive interfaces and provide targeted training to encourage employees to effectively use the tools. * Measure ROI – Track KPIs such as call resolution times, compliance errors, and customer satisfaction to justify costs and drive continuous improvements.CONCLUSION: AI AS THE FUTURE OF CUSTOMER SUPPORT EXCELLENCEAI-powered speech-to-text and GPT analysis are reshaping customer support byautomating tasks, providing real-time insights, and enhancing service quality.Whether in sales, technical support, or post-purchase care, these tools helpstreamline operations, identify key trends, and resolve issues faster.With solutions like OpenAI Whisper, Microsoft Azure Speech Services, and GoogleCloud Speech-to-Text, businesses can develop custom AI-driven solutionswithout building models from scratch. Byleveraging existing frameworks and APIs, companies can reduce development costswhile adapting AI-powered tools to their specific needs. However, challengesremain—accuracy limitations, compliance requirements, and the need for humanoversight must be carefully managed.By reducing manual workloads and delivering actionable insights, AI enablesbusinesses to respond more efficiently ,improve agent training, and strengthen customer relationships. Companies thatintegrate these technologies gain a competitive edge, optimizing bothoperational efficiency and customer experience.Supervised by Patryk Szczygło, R&D Lead at
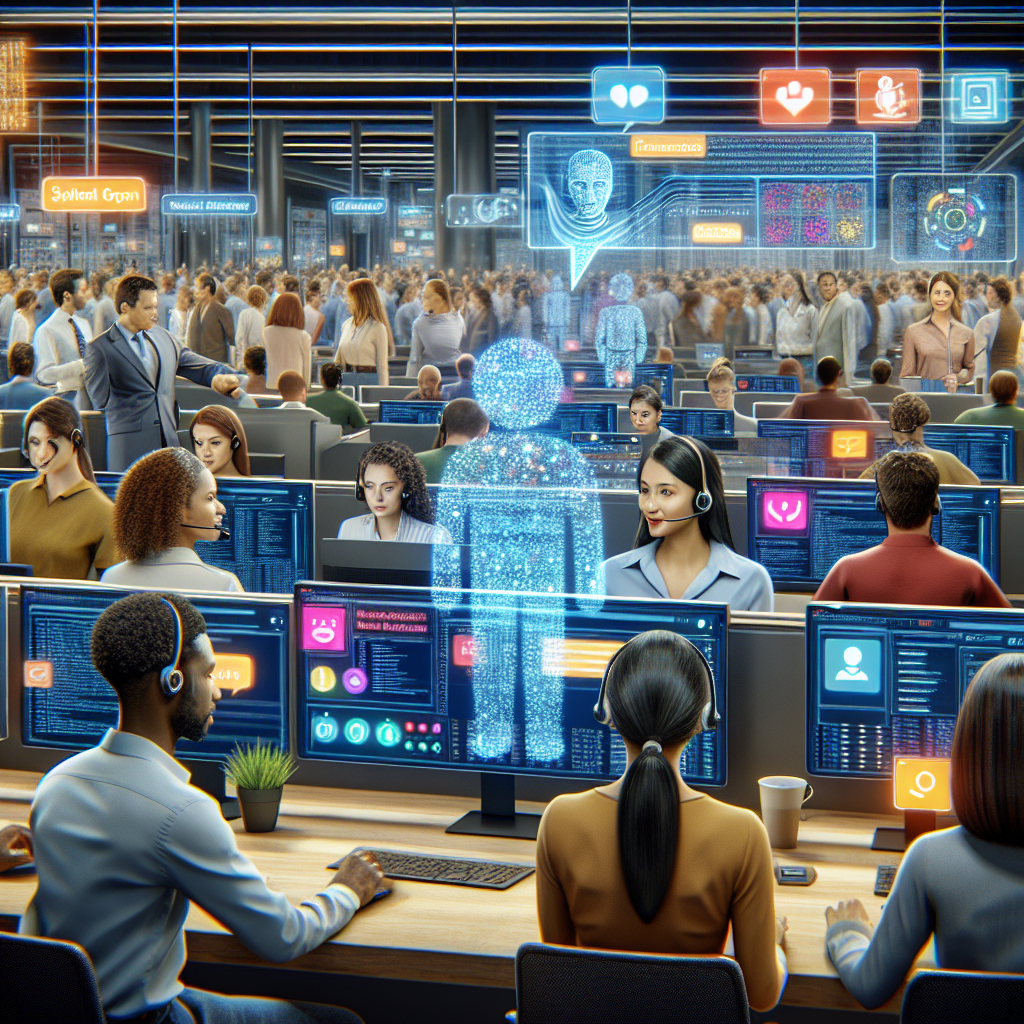
Speech-to-Text with GPT Sentiment Analysis Use Cases to Upgrade Helpdesk
Posted Date:
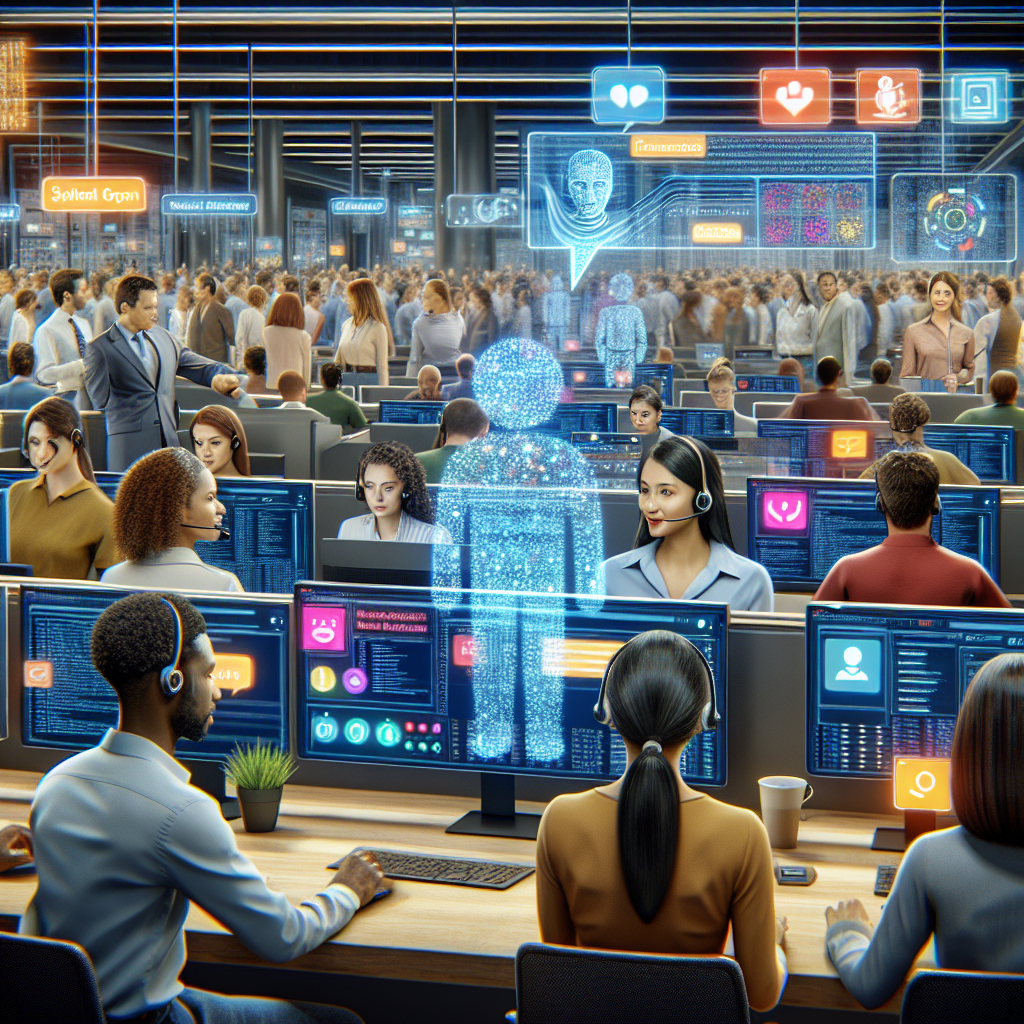